Testimonials
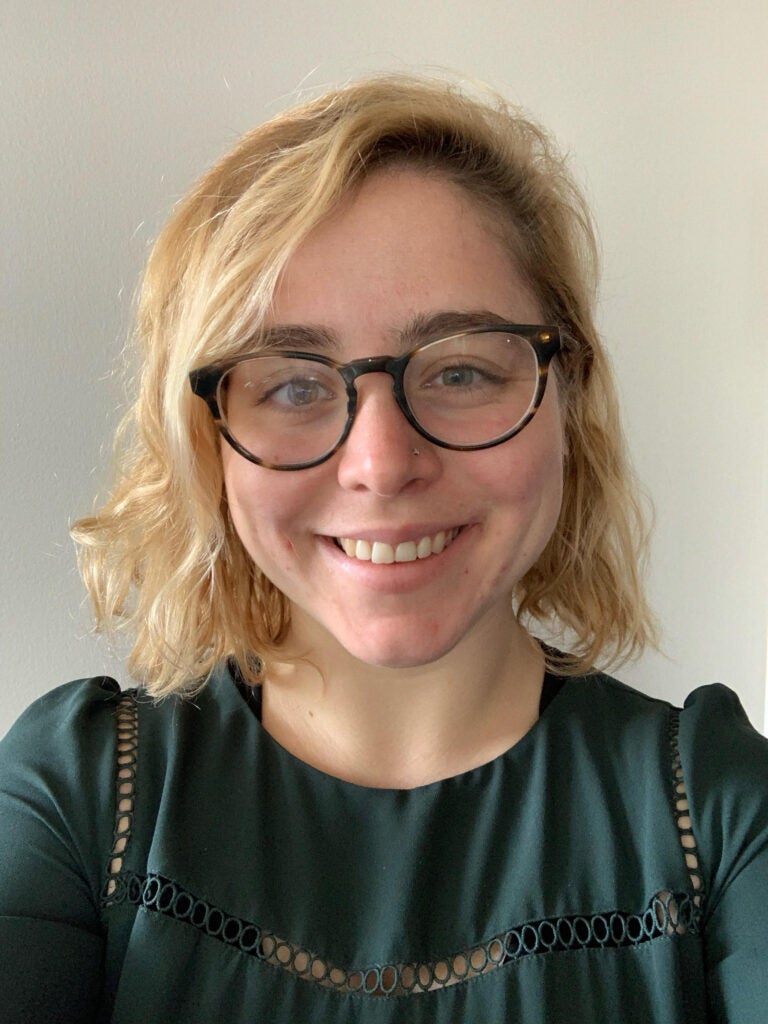
Senior Data Scientist | Leidos
Tell me about yourself / What did you do after graduation?
I am a researcher working out of the Leidos AI/ML Accelerator. Most of my work right now is within the field of Computer Vision, but I’ve also spent a good bit of time thinking about everything from RLWE homomorphic encryption to AI operationalization.
Why did you choose Georgetown?
I was an undergraduate mathematics major at Georgetown University. Upon graduation, I realized (very quickly) that if I was serious about practicing mathematics as a professional career, I would need at least a master’s degree (or many years of experience). The masters seemed like a quicker path to career happiness, and I had ties to the Georgetown community.
During undergrad, I had taken an advanced linear algebra class with Professor Ken Shaw. I went to see him three months after I graduated from my undergraduate degree to express my discontent working as an analyst at a fintech startup, which I’d very quickly realized was certainly not the direction I wanted to take my career. I knew, at that point, that as part of my professional life, I wanted to develop new mathematics and work closer to the cutting edge of AI/ML. However, I was lacking some of the necessary programming and applied mathematics skill sets – particularly around deep learning frameworks, modeling, and paper-reading/writing. Professor Shaw told me that participating in the MAST program would help me get closer to my goals, and I trusted him, both as a mentor and a friend. So, I only applied to Georgetown MAST, was accepted, and will forever be grateful to Professor Ken Shaw for helping me begin my professional career.
How did the MAST program prepare you for your career?
The program taught me the programming, applied mathematics, research, and theoretical backing necessary to successfully enter the workforce and meaningfully contribute towards my team’s research objectives. Most of my professors were also working professionals with excellent advice and years of experience in the field. Every class I took during my masters either provided a great deal of personal edification or professional wisdom.
What’s your advice for alums?
Your masters degree is only the beginning. Continue to both grow your deep understanding of mathematics/programming post-masters and your personal network – both will help you have a successful, fulfilling career (of which I am still only just starting).
Also, a lot of AI/ML modeling relies upon underlying hardware, sometimes a vacation does result in better model performance.
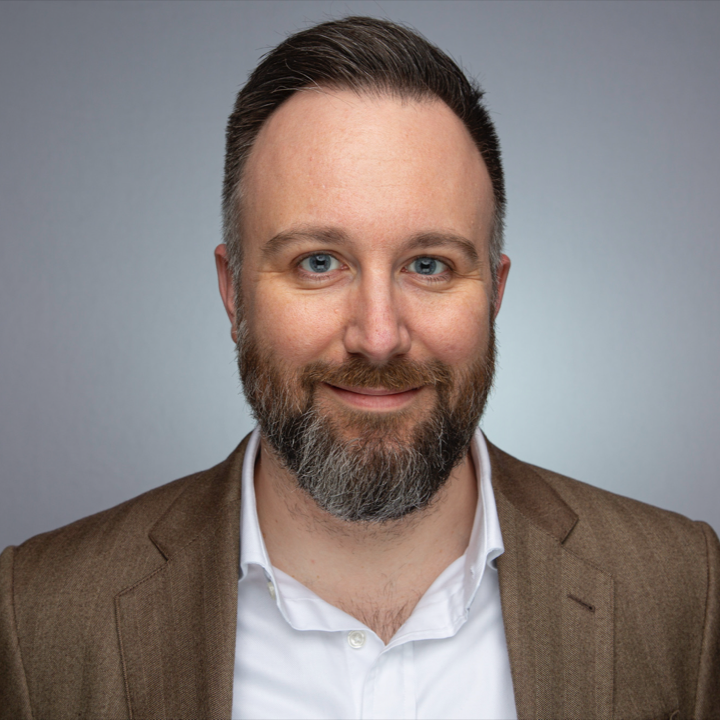
Senior Technology Staff Member |
In-Q-Tel
Tell me about yourself / What did you do after graduation?
After graduation, I held several interesting, if brief positions. I worked at a technology startup and as a legislative assistant in the House of Representatives, both part time and concurrently for several months. I then left to work as a statistician at the Science and Technology Policy Institute for two years. I left to be Director of Data Science at Impact Research, a small firm doing data science for auto safety. After two years there, I left for In-Q-Tel, a nonprofit at the nexus of venture capital, startup technology, and national security. I have been at In-Q-Tel since 2017. On the side, I started a PhD in Computational and Data Science at George Mason University since 2015. I am now a PhD candidate and hope to have my dissertation finished in 2022.
Why did you choose Georgetown?
Truly, it was circumstance. (But I am so happy I did it!) I had been a math minor in undergrad and was hoping to go into a PhD program in economics. Rather than taking more undergraduate math classes, I discovered that MAST would let me get a master’s degree. Its flexibility and not requiring a thesis was perfect for my goals at the time, even though my plans changed.
How did the MAST program prepare you for your career?
I cannot overstate how valuable a rigorous education in probability and statistics has been for me. In today’s world, so many people are getting into machine learning and data science, you need a way to differentiate yourself and add value that others can’t. Yes, you need to know how to program. But that isn’t a differentiator. Most people simply don’t have the background to engage with the broad swath of methods in use today. But my education allowed me to engage with all areas of machine learning and link them back to more basic principles. That gets me unstuck, find creative solutions, and get to deep issues faster than many of my contemporaries coming from different disciplines. And, frankly, MAST’s probability and statistics curriculum is so rigorous, I’ve found that I know more theory than many graduates of other masters-level statistics programs.
What’s your advice for alums?
Your education will leave you well-prepared for the technical aspects of a beginning career in data science. Lean into learning the soft human skills like empathy and communication, and you will be extremely well placed for the remainder of your carrer.
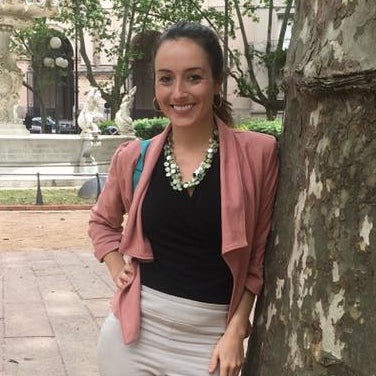
Statistics P.hD. Student | Carnegie Mellon University
Tell me about yourself / What did you do after graduation?
I did not have a strictly “mathy” background. My undergraduate degree is in international studies and Spanish, and then I spent several years abroad working in economic development (as a Peace Corps Volunteer in Ecuador, a Fulbright Scholar in Portugal, a Boren Fellow in Turkey, and as a J-PAL research associate in Mozambique). I had previously completed an MPA and MA-economics, so I had done my calculus and linear algebra sequence and some econometrics courses, but that was it. I wanted to pursue more rigorous quantitative tools while simultaneously working full time.
It took me three years to complete the degree part-time, and it was intense, but it paid off. After just one year in the program, people at my organization (the Inter-American Development Bank, or IDB) heard about my background through the grapevine. I was promoted as data scientist for a vice presidency at the IDB, where I worked on several awesome projects using predictive modeling and network analysis. Outside the IDB, I also led several courses on machine learning and coding in R, and I was twice a speaker at the R|Gov conference.
Now I’m a PhD student in statistics at Carnegie Mellon University, which is an ideal setting for combining statistical theory with useful applications in machine learning, industry, and public policy. I am so honored to be a Georgetown alum and thanks to my professors and the MAST team, I am living my dream.
Why did you choose Georgetown?
One, Georgetown’s MS-math/stats program is the perfect blend of theory and application. As its name suggests, the program is based on math. I always recommend math as the “starting point” for careers in data science or statistics: it’s a common foundation so we can communicate ideas precisely and in a common language. I learned to code in R mostly by doing, and I became fluent in visual tools such as Tableau at work. I have a relatively weaker background in computer science, although many of the tools are the same (such as numerical methods).
Two, I was looking for a program that would fit my full-time work schedule, and the evening class hours were perfect for me.
Three, Georgeotwn has an excellent overall academic reputation, and the department and its faculty certainly live up to its name. It was an honor to be a student there.
How did the MAST program prepare you for your career?
Certainly I had more job offers once I earned the MS degree, both within and outside of my organization. I wasn’t usually even looking for a job, but people in my network would hear about me. Within Georgetown, I also made lifelong friends and colleagues (both students and professors) and the alumni network is great for finding career and research opportunities.
Certainly, having gone to Georgetown helped me in pursuing a PhD. Most importantly, the professors were like family. I felt like my professors cared about me and they helped me catch up when my mathematical background was lacking.
What’s your advice for alums?
One, don’t be shy about re-learning old material. I often forget concepts and I have to review old notes (luckily I save everything, I’m very organized with my notes and assignments). It’s a good idea to review basics such as probability theory. I notice that the greatest NBA players are always practicing lay-ups on their own time (Ray Allen is one example), and the same idea applies to us all: we need to keep our foundations strong.
Two, help others. Learning math and statistics is a process. Other people will appreciate it so much if you share your knowledge and advice. Never pre-judge anyone as being “not smart” – that person was me! We all learn through persistence and practice, so I try to be genuine with those who were once in my shoes.